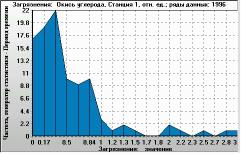 Figure 6. Ratio of modelled CO concentration to actual data (X-axis). Histogram of 4-times-a-day values over 1 year (Y-axis: occurrence per year). Monitoring station 1, year 1996. |
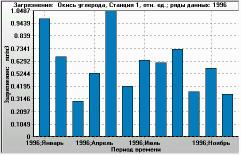 Figure 7. Monthly time series for the same ratio (plotted as Y-axis). X-axis: 1996, January to 1996, December. Monitoring station 1, year 1996. The filtering used was the same as in the histogram. |
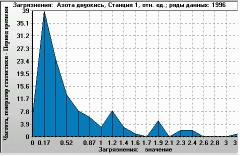 Figure 8. Ratio of modelled NO2 concentration to actual data (X-axis). Histogram of 4-times-a-day values over 1 year (Y-axis: occurrence per year). Monitoring station 1, year 1996. |
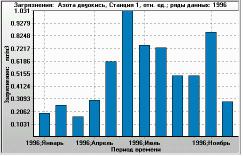 Figure 9. Monthly time series for the same ratio (plotted as Y-axis). X-axis: 1996, January to 1996, December. Monitoring station 1, year 1996.The filtering used was the same as in the histogram. |
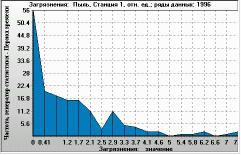 Figure 10. Ratio of modelled dust concentration to actual data for a single monitoring station (X-axis). Histogram of 4-times-a-day values over 1 year (Y-axis: occurrence per year). Monitoring station 1, year 1996. |
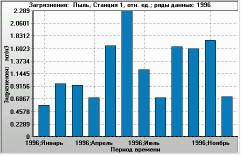 Figure 11. Monthly time series for the same ratio (plotted as Y-axis). X-axis: 1996, January to 1996, December. Monitoring station 1, year 1996. The filtering used was the same as in the histogram. |
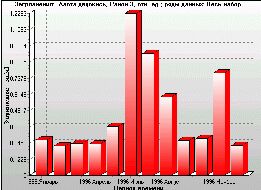 Figure 12. The ratio of NO2 model concentrations to measured data averaged over a city district (plotted as Y-axis). X-axis: 1996, January to 1996, December. City district 3, year 1996. Monthly averages of four-times-a-day ratios. |
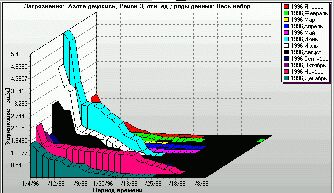 Figure 13. The ratio of NO2 model concentrations to measured data (plotted as Y-axis) averaged over city district 3 (monthly ranking of four-times-a-day ratios). X-axis: days of the respective month ranked highest concentration ratio first. |